Beyond Buzzwords: What is Natural Language Processing?
Naira Musallam, PhD • 12 Mar 2019
Out of the Weeds, Part II
In the first article of our Beyond Buzzwords series, we set out to demystify the meaning of machine learning, showcasing its relevance and practicality in the consumer insights space. This second installment is all about doing the same with natural language processing (NLP), also known as text analytics.
What is Natural Language Processing?
Natural language processing is widely acknowledged as a subfield of artificial intelligence. Its focus is on enabling computers to process and understand human languages. This allows NLP to perform functions like translations, semantic analysis, text classification, extraction, and summarization.
In practice, NLP relies on multiple disciplines, including computer science, computational power, statistics, and linguistics to understand human communications.
When we talk about NLP, we are usually referring to:
Content & topic categorization
The ability to organize a piece of text into meaningful themes or categories. It could be behaviors, products, or any organizing factor of importance to the end-user.
Speech-to-text and text-to-speech
Converting audio into written text and vice versa.
Document summarization
The ability to extract and create accurate textual summaries based on a large quantity of text.
Named Entity Recognition
The tagging of specific words (e.g. names, organizations, etc.) & parts of speech.
Sentiment analysis
Identifying the emotional reactions to include types of emotions, frequency, and intensity
How Does Natural Language Processing Work?
You might be asking yourself, this sounds great, but how does it work? How could a machine understand human language? What type of processes and algorithms are applied?
You might be surprised to know that accurate and actionable NLP outcomes often take hard work. Specifically, the work of computer scientists, engineers, linguists, and industry-specific experts, who do a significant amount of manual (and not-so-sexy) work to get the software to perform “artificially intelligent” tasks.
So, let’s say you have a million product reviews you want to analyze or 1,000 pages of text from consumer interviews, and you would like to extract the sentiments and/or understand the most popular topics
If the NLP software you are using is any good, the first step would be to clean the data. Just like organizing a messy excel sheet, NLP software combs through your results to clean the data- or at least reduce the “level of noise” to a minimum.
This critical first step is called pre-processing and it involves both “normalization” and “tokenization”.
Normalization
Normalization involves tasks like removing non-alphabetical characters, converting letters to lowercase, removing stop words (e.g. the, a, in, etc.), converting numbers to words, and stemming and lemmatization.
For further context, stemming and lemmatization work to reduce words to a common variant- the “stem” or “lemma”. The “stem” is the part of the word to which you add influential affixes such as -ed, -ize, mis, etc. Sometimes this results in words that are not actual words. The “lemma” is the base or dictionary form of the word.
Tokenization
Tokenization refers to segmenting the text into smaller chunks. This means paragraphs can be tokenized into sentences, and sentences into categories, sentiments, parts of speech, or parsing and then tagged the text with anything meaningful to the user (e.g. name recognition, sentiment, behaviors, etc.).
While there are readily available libraries with codes and algorithms that can perform the above tasks- if you are building your own lexical analysis and framework, you should tokenize your own text.
You might want to do this either because your framework is new, or you want to enhance the accuracy. Or, you could work with a software platform that has custom data sets relevant to your space, already built-in.
Tokenized text becomes a “golden dataset”, which is then used to “train” a statistical model, applied to any new text. This is where you may come across the term “supervised machine learning”.
Statistical Models for Natural Language Processing
Depending on what you are trying to achieve, there are a variety of statistical models that can be applied. These range from logistic regression models, to Support Vector Machine (SVM), or deep neural learning.
The type of statistical model you choose depends on the structure and complexity of your data and frankly is the result of continuous experimentation to increase the accuracy.
Hopefully, now, you feel a little better prepared to know what is available for your research.
But more importantly, be able to evaluate future solutions with a clearer understanding of the science and the technology supporting it.
Meet the author
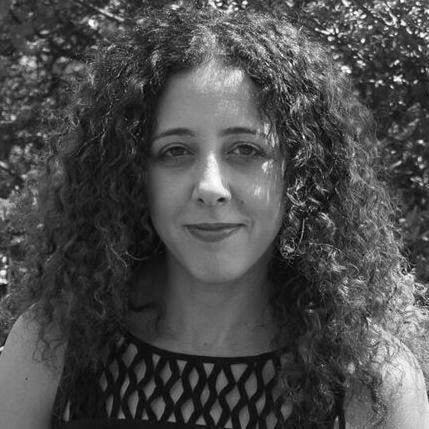
Naira Musallam, PhD
Generative AI is Here to Push the Limits of Market Research
While the technology of generative AI has been around for quite some time, it wasn’t until the introduction of Lindsay • 30 Sep 2024
Consumer Spending Outlook: Summer 2024
Despite most economic indicators showing the US economy is in good shape by historical standards, consumer confidence has begun to dip once again, Savannah Trotter • 11 Jul 2024
How to Write Effective Concept Testing Survey Questions
Your concept tests are only as good as the questions you include. When done correctly, Savannah Trotter • 07 Jun 2024
Concept Validation Strategies and Methods
When you're ready to validate a new idea, Naira Musallam, PhD • 22 May 2024
Data Privacy & Generative AI in Market Research
In the digital age, data privacy and security are paramount, especially when utilizing powerful generative AI tools, like our Lindsay • 05 Apr 2024
Unleashing the Power of Survey Pages with Randomization and Looping
When designing a The SightX Research Team • 27 Mar 2024
Meet the author
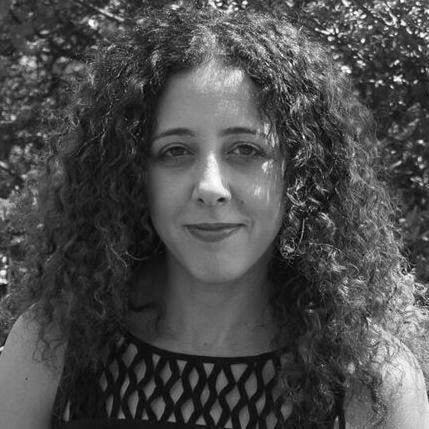
Naira Musallam, PhD
Ready to meet the next generation of consumer research technology?
The Future of Consumer Research