Demystifying Correlation in Research
Naira Musallam, PhD • 5 Dec 2024
Correlation is one of the most fundamental statistical concepts used in research, yet it is often misunderstood or oversimplified. Whether you’re exploring consumer behaviors, evaluating marketing campaigns, or assessing product performance, understanding correlation is key to uncovering relationships in your data. In this blog, we’ll explore correlation in depth, including how it’s measured, its types, and how tools like SightX can help you harness its power for impactful insights.
What is Correlation in Research?
Correlation is a statistical measure that indicates the strength and direction of a relationship between two variables. It helps researchers determine whether and how strongly variables are related, offering insights into patterns and associations in the data.
For example:
- High correlation: Sales of ice cream and temperature during summer months.
- Low correlation: Ice cream sales and stock prices.
Correlation Coefficient
The correlation between two variables is quantified using a correlation coefficient, often represented by the letter r. This value ranges from -1 to 1:
- 1: Perfect positive correlation (as one variable increases, the other increases).
- -1: Perfect negative correlation (as one variable increases, the other decreases).
- 0: No correlation (no linear relationship between the variables).
Measuring Correlation
There are several methods to measure correlation, depending on the type of data and relationship you’re analyzing.
1. Pearson Correlation
The Pearson correlation coefficient measures the linear relationship between two continuous variables. It assumes a normal distribution and is ideal for interval or ratio data.
Formula:
r=∑(xi−xˉ)(yi−yˉ)∑(xi−xˉ)2∑(yi−yˉ)2r = \frac{\sum (x_i - \bar{x})(y_i - \bar{y})}{\sqrt{\sum (x_i - \bar{x})^2 \sum (y_i - \bar{y})^2}}
2. Spearman’s Rank Correlation
This non-parametric method measures the strength and direction of a monotonic relationship (not necessarily linear) between two ranked variables.
3. Kendall’s Tau
Another non-parametric measure, Kendall’s Tau, assesses the strength of relationships between ordinal variables and is particularly useful for small sample sizes.
Negative Correlation
A negative correlation occurs when one variable increases while the other decreases.
Examples in Research
- As the price of a product increases, the quantity purchased often decreases (price elasticity).
- As time spent on manual processes decreases, operational efficiency increases.
Interpreting Negative Correlation
Negative correlations are not inherently bad. For instance, a business reducing customer complaints over time would see a negative correlation between time and complaint volume—an indicator of improvement.
Positive Correlation
A positive correlation occurs when both variables move in the same direction.
Examples in Research
- As advertising spend increases, sales revenue tends to increase.
- The longer a customer stays with a subscription service, the more likely they are to upgrade to premium plans.
Interpreting Positive Correlation
Positive correlations are often seen as favorable, but they can also indicate undesirable trends, such as increased production costs leading to higher retail prices.
What is a Correlation Matrix?
A correlation matrix is a table that displays the correlation coefficients for multiple variables at once. It is an essential tool for understanding complex datasets with numerous interrelated variables.
Why Use a Correlation Matrix?
- Quick Overview: Understand relationships across all variables in a dataset.
- Identify Patterns: Spot clusters of variables with high correlations.
- Preliminary Analysis: Use it as a starting point for deeper statistical analyses like regression.
Interpreting a Correlation Matrix
Each cell in the matrix shows the correlation coefficient between two variables. The diagonal typically shows 1s (as each variable is perfectly correlated with itself).
Example:
Variable A |
Variable B |
Variable C |
1.0 |
0.85 |
-0.45 |
0.85 |
1.0 |
-0.30 |
-0.45 |
-0.30 |
1.0 |
Platforms like SightX make it easy to generate and interpret correlation matrices visually.
Correlation and Causation
One of the most common pitfalls in research is confusing correlation with causation.
Correlation ≠ Causation
Just because two variables are correlated does not mean one causes the other. For example, an increase in ice cream sales correlates with an increase in drowning incidents, but this doesn’t mean ice cream causes drowning. Both are linked to a third variable: hot weather.
Testing for Causation
To establish causation, researchers must conduct experiments or use advanced statistical methods like regression analysis.
For an related post focused on understanding the differences between correlations, predictions, and causation click here.
Why Use Correlation?
Correlation is a versatile tool in research, offering several advantages:
1. Identifying Relationships
Correlation helps pinpoint associations between variables, guiding further analysis or hypothesis testing.
2. Simplifying Complex Data
With large datasets, correlation helps distill relationships, making data easier to interpret.
3. Supporting Decision-Making
Correlation insights inform strategies, whether in marketing, product development, or operational efficiency.
SightX Tools for Advanced Research
Platforms like SightX simplify correlation measurement by offering built-in analytics tools. Instead of calculating coefficients manually, SightX allows you to upload your data and visualize relationships effortlessly.
Harnessing the full potential of correlation requires robust tools. SightX offers an array of features that enable businesses to explore relationships and uncover actionable insights.
1. Regression Analysis
Regression goes beyond correlation to model the relationship between a dependent variable and one or more independent variables. This is particularly useful for predicting outcomes and identifying causal relationships.
Use Case: Predicting how changes in advertising spend affects sales.
2. Conjoint Analysis
Conjoint analysis helps businesses understand how customers value different product features by evaluating trade-offs.
Use Case: Identifying which product attributes drive purchase decisions.
3. T-Test
The T-test compares the means of two groups to determine if differences are statistically significant.
Use Case: Comparing customer satisfaction scores before and after a service upgrade.
4. Cross-Tab Analysis
Cross-tabulation analyzes relationships between categorical variables, offering insights into segmented data.
Use Case: Exploring how customer preferences vary by demographic group.
SightX integrates these tools into a seamless platform, making it easy for researchers to conduct advanced analyses and extract meaningful insights.
Conclusion
Understanding and leveraging correlation is essential for effective research. From identifying patterns to guiding strategic decisions, correlation offers a foundation for exploring relationships in data.
By using advanced tools like SightX, researchers can not only measure correlation but also dive deeper into regression, conjoint analysis, and other methodologies to uncover actionable insights. Ready to elevate your research? Explore how SightX can transform your data into decisions today!
Meet the author
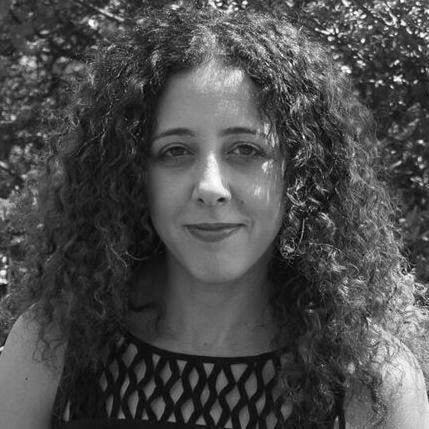
Naira Musallam, PhD
Ready to meet the next generation of consumer research technology?
The Future of Consumer Research